Success at the UTS Cross Faculty Collaboration Scheme 2022
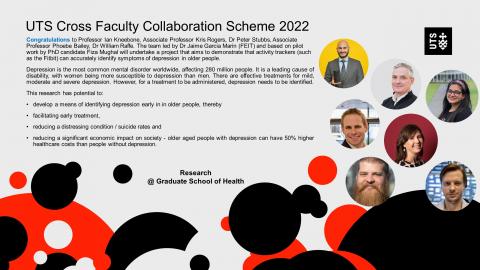
Congratulations to Professor Ian Kneebone, Associate Professor Kris Rogers, Dr Peter Stubbs, Associate Professor Phoebe Bailey, Dr William Raffe. The team led by Dr Jaime Garcia Marin (FEIT) and based on pilot work by PhD candidate Fiza Mughal will undertake a project that aims to demonstrate that activity trackers (such as the Fitbit) can accurately identify symptoms of depression in older people.
Depression is the most common mental disorder worldwide, affecting 280 million people. It is a leading cause of disability, with women being more susceptible to depression than men. There are effective treatments for mild, moderate and severe depression. However, for a treatment to be administered, depression needs to be identified.
This research has potential to:
- develop a means of identifying depression early in in older people, thereby
- facilitating early treatment,
- reducing a distressing condition / suicide rates and
- reducing a significant economic impact on society - older aged people with depression can have 50% higher healthcare costs than people without depression.
Project Details:
Aim:
This project aims to demonstrate that activity trackers (such as the Fitbit) can accurately identify symptoms of depression in older aged people.
Significance:
Depression is the most common mental disorder worldwide, affecting 280 million people . It is the leading cause of disability worldwide, with women being more susceptible to depression than men.
There are effective treatments for mild, moderate and severe depression. However, for a treatment to be administered, depression needs to be identified. in most cases, depression is often undiagnosed, which can lead to a poorer quality of life, episodes of self-harming and even suicide. Suicide is the fourth leading cause of death worldwide, with 700,000 people dying due to suicide every year. Rates of suicide are highest among males aged 70+ years, and depression is a contributing factor to suicide. Our project aims to identify depression in older people, which will allow for appropriate and effective interventions.
Background:
In a previous study, we (a group of collaborators from the Faculty of Engineering and IT and the Graduate School of Health) demonstrated that activity data from wearable trackers (such as the Fitbit) might be used to predict depressive tendencies in older aged people.
We developed a system architecture framework that optimises several processes such as data collection, data processing and data visualisation. More importantly, we developed a machine learning algorithm that can discriminate between depressed and non-depressed older adults based on activity data.
A small feasibility study on 12 older aged people demonstrated that activity measures such as: heart rate, daily activity, and sleep patterns, among others, can be indicative of depressive symptoms. Our study was also very successful in showing that it is feasible to use wearable technology in older aged people by identifying their motivations, barriers to adoption and preferences. This study however had limitations primarily around the sample size and the limited number of data points to train our machine learning algorithm.
Social Impact:
As part of the ageing process, the human body undergoes a series of changes that often leads to a decline in physical and cognitive functions. In some cases, these could turn into chronic and debilitating medical disorders such as diabetes, Alzheimer’s and dementia. This combined with losing a friend or loved one, can have a significant impact on the mental health of older aged people.
Depression can have devastating mental and physical consequences, which can complicate existing conditions or could lead to the development of new illnesses.
Studies have shown that depression could have a severe impact in eating habits, often resulting in either obesity or loss of appetite (the main cause of geriatric anorexia). It can also disturb sleep, with many people with depression Furthermore, depressed older aged people also report longer than normal reaction times. This inherently increases the hazards associated with carrying out daily life activities such as cooking, driving, self-medication, to name a few. In addition, it can increase falls, with an inability to appropriately regain balance, following a loss of balance.
Currently, 68% of adults over the age of 65 have none or very minimal knowledge of depression, and its consequences. Also, only 42% of those that experience depression seek help from a health professional.
Early detection and adequate treatment can counteract and prevent the above mentioned emotional and physical consequences, making this project a social imperative.
This project has the potential to reduce undiagnosed or reported mental health incidents in older aged people.
This research could be quintessential in reducing the medical costs associated with the treatment of severe depression, having a significant economic impact on modern society. Older aged people with depression often have 50% higher healthcare costs than people without depression .
Additionally, this may also reduce caregiver burden and dependence. Worldwide, this combined group (older aged adults with depression and their carers) will potentially consist of 173 million people in 2022 alone, with numbers rising to 253 million by 2025.
Being able to detect depression in a timely manner through the use could be instrumental in reducing the high rates of suicide deaths among the elderly. It’s been reported that individuals aged 65 and older account for 20% of all suicide deaths, with males being particularly vulnerable.
Team:
- Fiza Tariq Mughal FEIT.School of Computer Science
- A/Prof Kris David Rogers GSH.Graduate School of Health
- A/Prof Phoebe Bailey GSH.Clinical Psychology
- Dr Peter William Stubbs GSH.Physiotherapy
- Dr William Luke Raffe FEIT.School of Computer Science
- Prof Ian Kneebone GSH.Clinical Psychology
- Dr Jaime Andres Garcia FEIT.School of Computer Science